

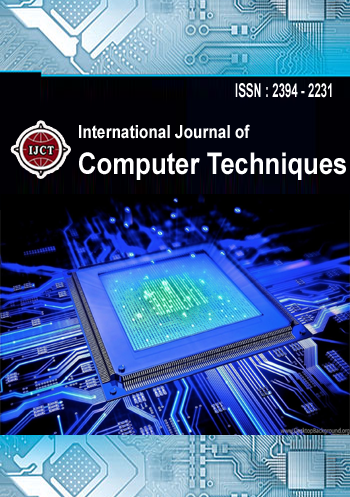
Paper Title : MARKET BASKET ANALYSIS
ISSN : 2394-2231
Year of Publication : 2022



MLA Style: MARKET BASKET ANALYSIS " Mr.S.Sambasivam,N.Nandhini " Volume 9 - Issue 2 International Journal of Computer Techniques (IJCT) ,ISSN:2394-2231 , www.ijctjournal.org
APA Style: MARKET BASKET ANALYSIS " Mr.S.Sambasivam,N.Nandhini " Volume 9 - Issue 2 International Journal of Computer Techniques (IJCT) ,ISSN:2394-2231 , www.ijctjournal.org
Abstract
Market Basket Analysis or MBA is a field of modelling ways grounded upon the proposition that if you buy a certain group of particulars, you're further (or lower) likely to buy another group of particulars. MBA includes determination and vaticination client’s geste grounded on expenditure pattern of former guests. MBA is applied not only for retail industries but also for a great number of other industries. There are studies which point to MBA and contribute to adding inflows in hospices operation by offering more seductive fresh services for new and regular guests. MBA grounded on multidimensional log it model was used to conduct a study Request handbasket analysis is to make a choice of purchasing, sailing or power of stocks in an equity request. Data booby-trapping ways insure high perfection of vaticination of stock price movement. In this thesis using MBA for perfecting styles of arranging products on store shelves was linked. Analysis of the most frequent guests’ deals was performed. In this design, Request handbasket vaticination, i.e., supplying the client a shopping list for the coming purchase according to her current requirements, is one of these services. Current approaches aren't able of landing at the same time the different factors impacting the client’s decision processcooccurrence, sequentuality, periodicity and recurrency of the bought particulars. To this end, this design defines a pattern Temporal Annotated Recurring Sequence (Seamen) suitable to capture contemporaneously and adaptively all these factors. We define the system to prize TARS and develop a predictor for coming handbasket named TBP (TARS Based Predictor) that, on top of TARS, is suitable to understand the position of the client’s stocks and recommend the set of utmost necessary particulars. By espousing the TBP the supermarket chains could crop acclimatized suggestions for each individual client which in turn could effectively speed up their shopping sessions
Reference
[1] R. Guidotti, A. Monreale, S. Ruggieri, F. Turini et al., “A survey of methods for explaining black box models,” ACM Computing Surveys (CSUR), vol. 51, no. 5, p. 93, 2018. [2] Y.-A. de Montjoye, E. Shmueli, S. S. Wang, and A. S. Pentland, “openpds: Protecting the privacy of metadata through safeanswers,” PloS one, vol. 9, no. 7, p. e98790, 2014. [3] M. Vescovi, C. Perentis, C. Leonardi, B. Lepri, and C. Moiso, “My data store: toward user awareness and control on personal data,” in Ubicomp. ACM, 2014, pp. 179–182. [4] K. Christidis et al., “Exploring customer preferences with probabilistic topic models,” in ECML-PKDD, 2010. [5] Adomavicius, G., Tuzhilin, A.: Toward the next generation of recommender systems: a survey of the stateofthe-art and possible extensions, Knowledge and Data Engineering, IEEE Transactions, vol. 17, 734—749 (2005). [6] B. Goodman and S. Flaxman. Eu regulations on algorithmic decision-making and a right to explanation. In ICML workshop on human interpretability in machine learning (WHI 2016), New York, NY. http://arxiv. org/abs/1606.08813 v1, 2016. [7] S. Wachter, B. Mittelstadt, and L. Floridi. Why a right to explanation of automated decision-making does not exist in the general data protection regulation. International Data Privacy Law, 7(2):76–99, 2017. [8] G. Comand`e. Regulating algorithms regulation? first ethico-legal principles, problems, and opportunities of algorithms. In Transparent Data Mining for Big and Small Data, pages 169–206. Springer, 2017. [9] A. Weller. Challenges for transparency. arXiv preprint arXiv:1708.01870, 2017. [10] J. M. Hofman, A. Sharma, and D. J. Watts. Prediction and explanation in social systems. Science, 355(6324):486–488, 2017. [11] J. Huysmans, K. Dejaeger, C. Mues, J. Vanthienen, and B. Baesens. An empirical evaluation of the comprehensibility of decision table, tree and rule based predictive models. Decision Support Systems, 51(1):141– 154, 2011. [12] A. A. Freitas. Comprehensible classification models: a position paper. ACM SIGKDD explorations newsletter, 15(1):1–10, 2014. [13] D. Pedreshi, S. Ruggieri, and F. Turini. Discrimination-aware data mining. In Proceedings of the 14th ACM SIGKDD international conference on Knowledge discovery and data mining, pages 560–568. ACM, 2008. [14] F. Pasquale. The black box society: The secret algorithms that control money and information. Harvard University Press, 2015. [15] H. Lakkaraju, J. Kleinberg, J. Leskovec, J. Ludwig, and S. Mullainathan. The selective labels problem: Evaluating algorithmic predictions in the presence of unobservables. In Proceedings of the 23rd ACM SIGKDD International Conference on Knowledge Discovery and Data Mining, pages 275–284. ACM, 2017. [16] D. McSherry. Explanation in recommender systems. Artificial Intelligence Review, 24(2):179–197, 2005. [17] S. Krening, B. Harrison, K. M. Feigh, C. L. Isbell, M. Riedl, and A. Thomaz. Learning from explanations using sentiment and advice in rl. IEEE Transactions on Cognitive and Developmental Systems, 9(1):44–55, 2017
Keywords
— Data Mining, Market Basket Analysis, Temporal Annotated Recurring Sequence.