

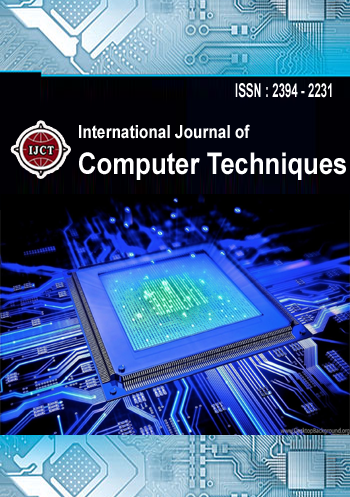
Paper Title : IDENTIFICATION OF COVID-19 FUTURE FORECASTING
ISSN : 2394-2231
Year of Publication : 2022



MLA Style: IDENTIFICATION OF COVID-19 FUTURE FORECASTING " Dr.E.K VellingiriRaj, Mohamed Ali Jinna.M " Volume 9 - Issue 2 International Journal of Computer Techniques (IJCT) ,ISSN:2394-2231 , www.ijctjournal.org
APA Style: IDENTIFICATION OF COVID-19 FUTURE FORECASTING " Dr.E.K VellingiriRaj, Mohamed Ali Jinna.M " Volume 9 - Issue 2 International Journal of Computer Techniques (IJCT) ,ISSN:2394-2231 , www.ijctjournal.org
Abstract
The Spread of COVID-19 in the whole world has endangered the mankind. The resources of irrefutably the greatest economies are stressed due to the immense infectivity and infectiousness of this affliction. The capacity of ML models to appraise the amount of impending patients affected by COVID-19 which is at this point considered as a potential risk to humankind. In particular, five standard guaging models, specifically LR, LASSO, SVM, ES, have been used in this survey to check the sabotaging factors of COVID-19.Three sorts of assumptions are made by all of the models, similar to the amount of as of late tainted cases, the amount of passing, and the amount of recoveries But in the can't anticipate the exact result for the patients. To overcome the issue, proposed procedure using the long transient memory (LSTM) predict the amount of COVID-19 cases in next 10 days ahead and effect of preventive appraisals like social disconnection and lockdown on the spread of COVID-19.
Reference
Alsaeedy, A. A. R., and Chong, E. (2020). Recognizing Regions at Risk for Spreading COVID-19 Using Existing Cellular Wireless Network Functionalities. IEEE Open Journal of Engineering in Medicine and Biology, 1-1. 2. Sear, R. F., Velasquez, N., Leahy, R., Restrepo, N. J., El Oud, S., Gabriel, N., … Johnson, N. F. (2020). Assessing COVID-19 substance in the web based prosperity appraisal war using AI. IEEE Access, 1-1. 3. Hu, S., Gao, Y., Niu, Z., Jiang, Y., Li, L., Xiao, X. … Yang, G. (2020). Miserably Supervised Deep Learning for COVID-19 Infection Detection and Classification from CT Images. IEEE Access, 1-1. 4. Zhang, Y., Li, Y., Yang, B., Zheng, X., and Chen, M. (2020). Danger Assessment of COVID-19 Based On Multisource Data from a Geographical View. IEEE Access, 1-1. 5. Abdel-Basset, M., Mohamed, R., Elhoseny, M., Chakrabortty, R. K., and Ryan, M. (2020). A blend COVID-19 disclosure model using a further evolved marine tracker's estimation and a situating based assortment decline strategy. IEEE Access, 1-1. 6. F. Petropoulos and S. Makridakis, "Guaging the original Covid Corona virus," Plos one, vol. 15,no. 3, p. e0231236, 2020. 7. G. Grasselli, A. Pesenti, and M. Cecconi, "Essential thought use for the Coronavirus discharge up in lombardy, italy: early experience and guess during an emergency response," Jama, 2020. 8. C. P. E. R. E. Novel et al., "The epidemiological characteristics of an episode of 2019 novel Covid diseases (Coronavirus) in china," Zhonghua liu xing bing xue za zhi= Zhonghua liuxingbingxue zazhi, vol. 41, no. 2, p. 145, 2020. 9. Y. Grushka-Cockayne and V. R. R. Jose, "Joining assumption extends in the m4 competition," International Journal of Forecasting, vol. 36, no. 1, pp. 178-185, 2020. 10. N. C. Mediaite. Harvard teacher sounds alert on 'likely' Covid pandemic: 40% to 70% of world could be corrupted for the current year. Gotten to on 2020.02.18.
Keywords
— IDENTIFICATION OF COVID-19 FUTURE FORECASTING.